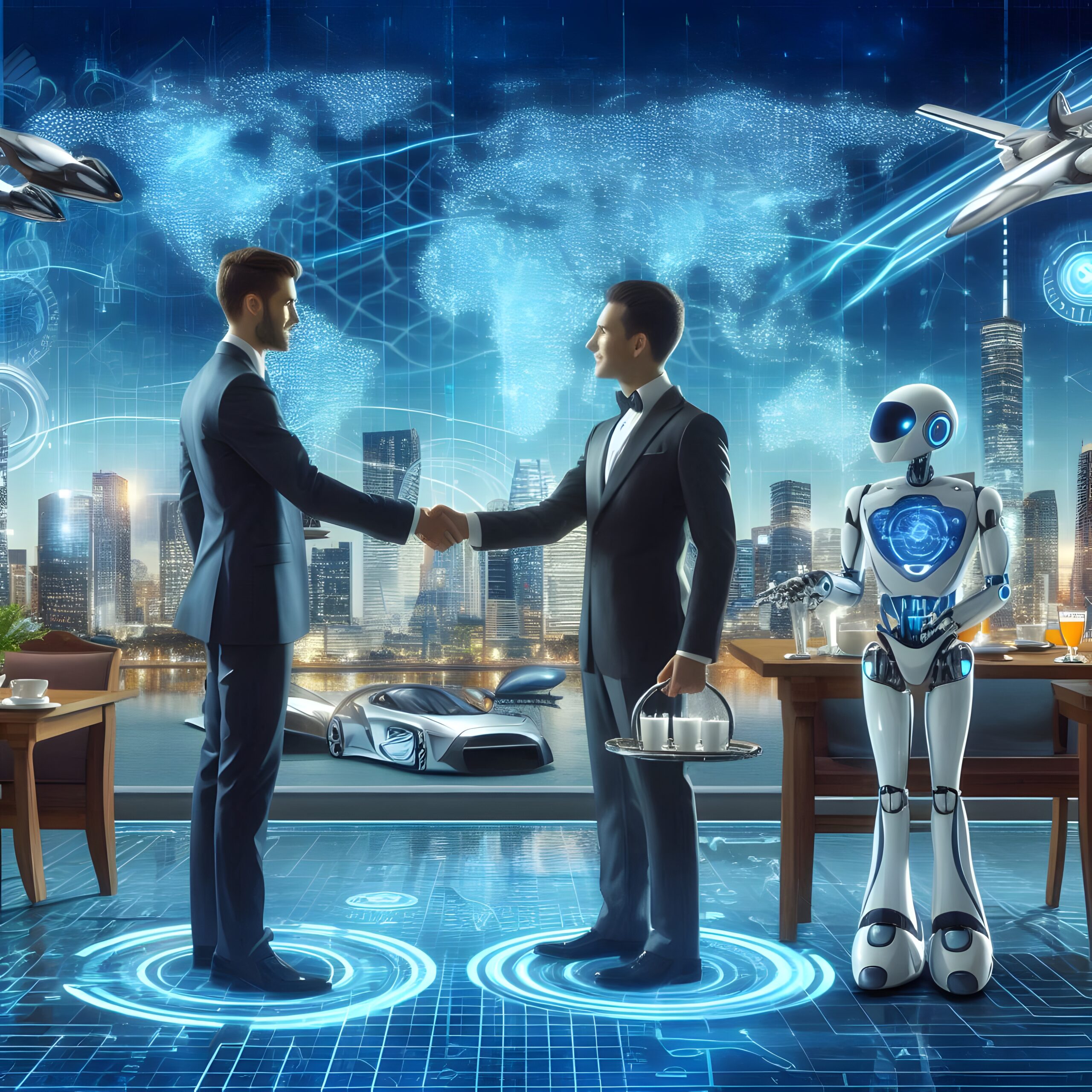
The History and Evolution of AI
Artificial Intelligence (AI) has rapidly transformed from a speculative idea into a powerful tool driving technological advancement in many fields. From its inception in the mid-20th century to its widespread applications today, AI has gone through several phases of growth and development. Understanding the history and evolution of AI is critical for anyone in the tech field, as it provides valuable context for the technologies shaping our future. This blog will take you through AI’s journey, its technical milestones, and the key factors influencing its progress up to 2024.
The Beginnings of AI: A Conceptual Foundation
The Birth of AI (1940s–1950s)
The foundation for AI was laid during World War II, when British mathematician Alan Turing proposed the idea of machines that could simulate any process of mathematical reasoning. His 1950 paper, “Computing Machinery and Intelligence,” introduced the famous Turing Test, a method to determine whether a machine could exhibit human-like intelligence.
Simultaneously, John von Neumann developed the architecture for modern computers, which allowed machines to store and process instructions—crucial for future AI developments. The 1956 Dartmouth Conference, organized by John McCarthy, is often considered the official birth of AI as an academic field. It was here that the term “Artificial Intelligence” was coined.
The Symbolic Era (1950s–1970s)
The initial approach to AI was based on symbolic reasoning, where machines used predefined rules to simulate intelligent behavior. Early AI programs, such as the Logic Theorist and General Problem Solver, were designed to manipulate symbols and perform logical reasoning. These programs were successful in solving simple problems but struggled with more complex, real-world scenarios.
Researchers in this era believed that AI could mimic human intelligence by following logical rules. The hope was that given enough time and computing power, AI would eventually reach human-level intelligence. However, these early methods proved inefficient for dealing with the uncertainties and ambiguities of the real world.
The AI Winter: Challenges and Setbacks (1970s–1980s)
By the 1970s, the limitations of symbolic AI became apparent. The high expectations set by early researchers led to disappointment as progress stalled. AI Winter refers to periods of reduced funding and interest in AI research due to unmet promises and slow advancements.
One of the major challenges was the combinatorial explosion, where the number of possible solutions increased exponentially with problem complexity. AI systems based on predefined rules struggled to cope with this, leading to a bottleneck in the development of intelligent systems. Governments and organizations began to pull back funding, believing that AI had limited potential.
The Emergence of Machine Learning (1980s–1990s)
AI saw a resurgence in the 1980s with the advent of machine learning (ML), a paradigm shift from rule-based systems to data-driven approaches. Instead of explicitly programming machines to perform tasks, researchers began to focus on creating algorithms that allowed computers to learn from data.
In particular, artificial neural networks gained attention. These models, inspired by the structure of the human brain, allowed computers to recognize patterns in data. While early neural networks had limited success, the groundwork was laid for future deep learning advancements.
The rise of expert systems, which used knowledge bases and inference engines to simulate human expertise in specific domains, also marked a new era of AI. These systems were successfully used in fields such as medical diagnosis and finance, though they were still limited by their dependence on human input for knowledge encoding.
AI Enters the Modern Era: Data and Deep Learning (2000s–2010s)
Deep Learning and Big Data
Deep learning, a subset of machine learning, became the dominant technique for AI research during this time. Deep learning algorithms, which rely on neural networks with multiple layers, began to outperform traditional machine learning models in tasks such as image recognition, speech processing, and natural language understanding.
Companies like Google, Facebook, and Microsoft invested heavily in AI research, leading to the development of AI-powered tools like Google Translate, Alexa, and self-driving cars. These advancements were fueled by the availability of large datasets (big data) and Graphics Processing Units (GPUs), which provided the computational power required for deep learning.
A critical milestone during this period was the success of AlphaGo in 2016. Developed by DeepMind, AlphaGo defeated the world champion Go player using a combination of deep learning and reinforcement learning. This event was a breakthrough in AI, as Go was considered an extremely complex game requiring intuition and creativity.
AI in Everyday Life
By the late 2010s, AI had infiltrated many aspects of daily life. From virtual assistants like Siri and Google Assistant to recommendation engines on Netflix and Amazon, AI-powered systems were shaping user experiences. In healthcare, AI began to assist with tasks such as medical image analysis, while autonomous vehicles started to show potential in reshaping the transportation industry.
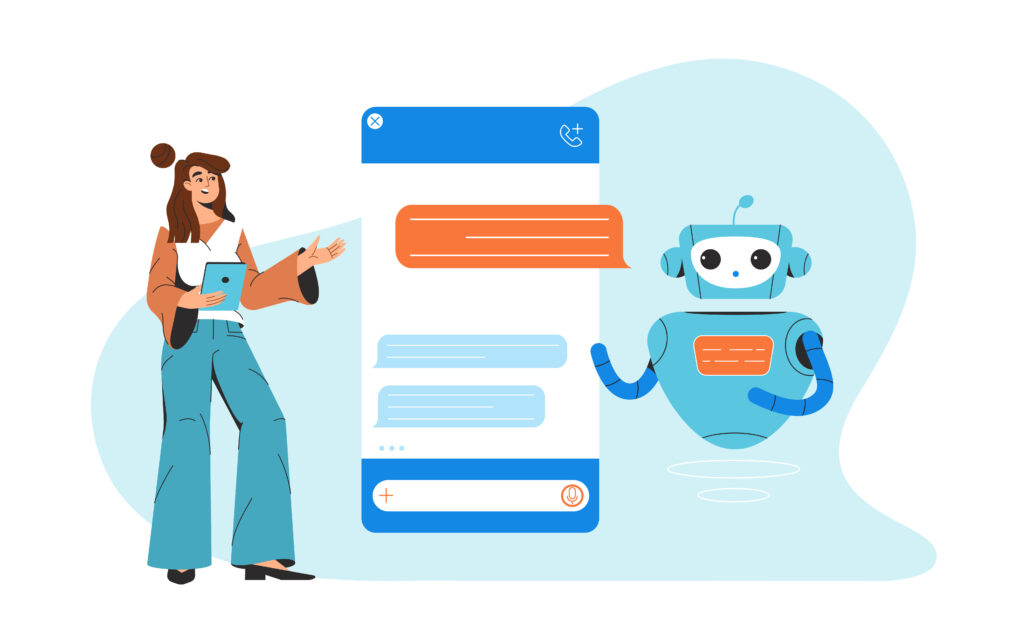
Chatbot technology. Flat woman chatting and communicating with artificial intelligence. Smartphone interface with chat bot dialog message icons at screen. AI robot assistant, online customer support.
AI’s role in business was also expanding, with predictive analytics, chatbots, and automation driving efficiency across sectors. Companies increasingly realized the value of AI in improving decision-making, customer experience, and operational efficiency.
AI in 2024: Current Trends and Future Prospects
Reinforcement Learning and Autonomous Systems
In 2024, reinforcement learning (RL) remains one of the most promising areas of AI research. RL involves training AI agents to interact with an environment and learn through trial and error. This approach has been instrumental in developing AI for robotics, self-driving cars, and complex game-playing.
Companies like Waymo and Tesla continue to push forward with autonomous driving, while AI-driven robots are being deployed in logistics, manufacturing, and even healthcare. The application of reinforcement learning in these areas has allowed for more adaptable and intelligent systems capable of handling real-world challenges.
Generative AI and Natural Language Processing (NLP)
Another major trend in 2024 is the rise of Generative AI and advancements in Natural Language Processing (NLP). Tools like OpenAI’s GPT-4 and Google’s BERT have set new benchmarks for language generation and comprehension, enabling AI to write articles, generate code, and create content that is difficult to distinguish from human output.
Generative AI is not limited to text; AI models now generate high-quality images, videos, and even music. These advancements have created new opportunities for content creators and businesses looking to automate creative tasks.
AI Ethics and Governance
As AI becomes more powerful, discussions around AI ethics and governance have become more critical. Issues such as algorithmic bias, data privacy, and the impact of AI on employment are being actively debated in 2024. Governments and tech organizations are working to establish ethical guidelines and regulations to ensure responsible AI development.
For instance, the European Union’s AI Act aims to regulate high-risk AI applications, ensuring transparency and accountability in AI systems. Similarly, major tech companies are forming AI ethics boards to oversee the ethical implications of their AI products.
Practical Steps for Implementing AI in 2024
1. Understand the Data
AI models are only as good as the data they are trained on. Ensure you have access to high-quality, well-labeled datasets relevant to your problem domain. For businesses, investing in data collection and cleaning processes is crucial for building accurate models.
2. Leverage Pre-Trained Models
In 2024, many companies can benefit from pre-trained models provided by platforms like Google Cloud AI, Microsoft Azure, and AWS AI. These models, trained on large datasets, can be fine-tuned for specific tasks, reducing the time and resources needed to develop AI systems from scratch.
3. Focus on Interpretability
As AI becomes more pervasive, the need for interpretability has grown. Make sure your AI models are interpretable and explainable, especially if they are used in high-stakes domains like healthcare or finance.
Conclusion
The history and evolution of AI demonstrate its transition from speculative theory to one of the most transformative technologies of our time. From the symbolic AI of the 1950s to today’s deep learning systems, AI has seen remarkable progress, with each era building on the discoveries of the last.
As we move into 2024, the opportunities presented by AI continue to expand, driven by advancements in reinforcement learning, NLP, and generative AI. However, along with these opportunities come ethical and governance challenges that must be addressed.
For businesses and individuals looking to harness the power of AI, the key lies in understanding its past, staying informed on current trends, and adopting best practices for implementation. As AI evolves, those who embrace it with a clear strategy will be well-positioned to lead in the digital age.